Look up “data governance” and you’ll probably find a definition filled with buzzwords that, at first glance, don’t say much.
With that being said, I too would like to define data governance in my own words.
However, before I do that, let me specify the primary downside of a lack of data governance across each stage of the data lifecycle.
Why you need data governance
Data collection
When data governance is not in place, naming conventions are not uniform and data is collected from disparate sources without predefined use cases, leading to data bloat and a lack of trust in data.
Data storage
Without data governance, increasingly large volumes of data are stored in multiple places, preventing organizations from maintaining a central repository, which is easier to manage in terms of access control.
Data consumption
Making accurate data accessible in the right tools for analysis and activation is a perpetual challenge when organizations are lacking data governance. This becomes a bottleneck for go-to-market (GTM) teams.
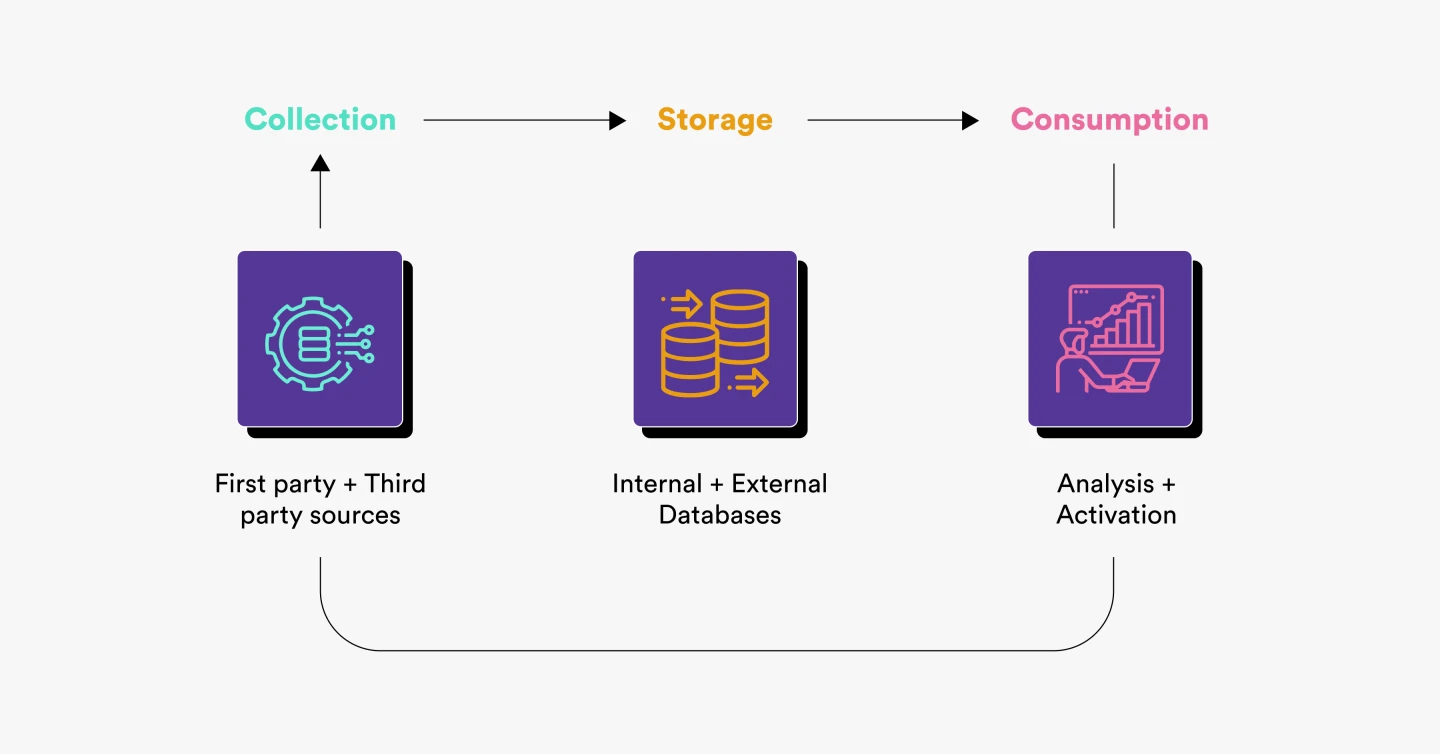
What is data governance?
So then, what exactly is data governance?
Data governance is a set of practices implemented to ensure data that is collected, stored, and made available for consumption is useful.
And for data to be useful, it needs to be uniform, complete, accurate, and accessible.
In this article, you will learn some measures that organizations can implement to put data governance into practice across every stage of the data lifecycle.
Governing data collection
Data is collected from a variety of sources with each source being either first-party (websites and apps powered by proprietary code) or third-party (external tools and APIs).
When it comes to data governance, it’s important to understand who decides what to collect, why certain data is collected, and who implements the collection process.
The following data governance best practices can be implemented at the time of data collection.
Get stakeholders to document how they intend to utilize data
Anyone who has been through the planning process of implementing data collection can attest to the lure of collecting every piece of data (all types of data, from all sources) long before even figuring out what the data will help them achieve. Collecting data without knowing how it will be used, what questions it will help answer, or what workflows it will help build or improve can lead to severe ramifications for teams.
However, stakeholders from marketing, sales, or customer success teams who plan and document what data they need for what purposes have more skin in the game as they understand the importance of collecting data that is accurate and usable.
Invest in tools to collect high-quality data and foster collaboration
Data quality tools come in many shapes and sizes, as different categories of tools solve different data quality problems.
Depending on the size of your organization and the scope of data workloads, the following categories of tools can help collect and maintain high-quality data.
- Behavioral data collection tools that have governance capabilities
- Testing, transformation, and observability tools
- Discovery and cataloging tools
While tools are important, getting caught up in lengthy evaluation processes is far too common. It helps to keep in mind that tools alone cannot solve problems unless they are implemented properly and used effectively by all stakeholders. In that case, make sure you either hire data consultants to help you in the implementation process or consider solutions that offer a strong customer success experience — like Y42.
Governing data storage
Data can be stored in a lot of places internally (in databases, data warehouses and lakes, or spreadsheets) and externally (in the data stores of third-party tools).
Who decides where to store the data and in what format? Should data stored in external systems be replicated in internal data storage?
There are no universally right answers to these questions. These are all highly subjective and what works for one organization might not work — or be feasible — for another.
However, keeping in mind that data needs to be useful, organizations must take the following steps to reduce redundancy while also ensuring the availability of the relevant data that teams need.
Don’t store data for the sake of storing it
Data teams typically set up a data warehouse to store all the data needed for analytics purposes. This includes replicas of production databases as well as data collected from both first-party and third-party sources.
However, what’s meant to serve the organization can very quickly become a bottleneck — like a data warehouse that is treated as a data dump.
Data teams must collaborate with business teams to understand whether raw data from certain sources needs to be warehoused for future use cases or not.
Sometimes, it’s enough to make data available in certain tools without storing a copy of it in the warehouse — this is especially true for event data that is primarily used to power automated workflows or perform analyses in external tools that store a copy of the data anyway.
Ensure that stored data is usable
As mentioned earlier, data is useful when it’s:
- Uniform
- Complete
- Accurate
- Accessible
But even for useful data to be usable — for analysis and activation purposes — it often needs to be cleaned and transformed.
And for data to be cleaned and transformed, data teams need to know where exactly the data will be consumed and for what purpose: analysis or activation.
Governing data consumption
The whole point of collecting and storing data is to enable people across teams to consume the data for analysis (to derive insights and inform decisions) and activation (to build personalized data-powered experiences).
Who can access the data warehouse? How is data made available in the tools business teams use for analysis and activation?
Organizations must invest in measures to ensure that everybody who needs data has access to it wherever they need it, while also ensuring that any misuse of data doesn’t go unnoticed. Here’s how they can start.
Make data accessible to the right people
There are two ways data can be made accessible:
- Give people access to the dataset
- Make the necessary data accessible in the tools where data is consumed
Organizations need to take measures to ensure that only the right people can access whatever data they need, wherever they need it.
This, of course, is easier said than done, and organizations need to invest in processes to ensure the following:
- Enable people to quickly find out what data is available and where — implementing a data catalog can help you achieve this.
- Make relevant data available in external tools where it’s consumed by business teams.
- Use tools to monitor the use of data assets to prevent unwarranted data sharing with external parties.
Making data accessible to the right people is a tiny but important step toward making data democratization a reality.
Keep security and compliance top of mind
Sound data governance should always include investing time in implementing best practices to keep your customer data secure, as well as adhering to privacy regulations.
In no particular order, organizations need to take strong measures for the following:
- Masking of PII data where it’s not needed or where it would lead to non-compliance.
- Revoking access to data assets once they stop being utilized.
- Ensuring financial data is not treated the same as, say, product-usage data.
It’s easy to turn a blind eye to these at the earlier stages of the data journey when becoming “data-driven” is a priority, but it’s definitely not worth the consequences of being in violation of data protection laws like the GDPR.
Conclusion
By no means is this a one-size-fits-all guide and there are, of course, other measures companies can take to make data governance a priority across the board.
I’d like to conclude by summarizing the six measures organizations can take to roll out their own data governance practice:
- Getting stakeholders involved: Ask them to describe how they intend to use the data they ask for.
- Investing in the right tools: Ensure data quality, identify issues, and enable collaboration between teams.
- Warehouse what’s needed: Only store data that is used to answer questions or power experiences.
- Make data usable: Useful data needs to be transformed to become usable.
- Make data accessible: Usable data needs to be made available where teams wish to consume it to perform their own analysis or drive actions.
- Security and compliance: Make these top of mind sooner rather than later.
Want to make data governance a priority? Book a call with Y42’s data experts to learn more about how to start implementing a data governance practice in your organization.
Category
In this article
Share this article