Introduction
When I first started as a data engineer, I worked on a DevOps-focused team. While it wasn’t exactly what I wanted to be doing in my first role, it taught me a lot. Now looking back, if I hadn’t worked in that type of role then, I probably wouldn’t have the experience I have as an analytics engineer today.
Now, working as an analytics engineer, I focus on something called DataOps. While this may sound similar to DevOps, they are very different. DevOps focuses on software as the product while DataOps focuses on producing high-quality data. For those focusing on DataOps, data is the product!
In my time working as a DevOps data engineer, I supported software engineers making code changes to our web application. I focused on testing changes in the UI after each deployment rather than looking into the specifics of the data. Not once did I check on the number of rows in a table or if values in a field were populated. Instead, I made sure no errors were being thrown on the backend.
As an analytics engineer, any time I make a code change or push something to production, I need to focus on the metadata- or the data about the data. This involves writing validation queries to ensure things like row count, column count, and distribution of values look as they did before I pushed a change. Or, if I want them to look different than they did before, they reflect those changes!
Although DevOps and DataOps sound similar, they serve two different purposes. In this article we will dive deeper into the differences, touching on the product they aim to serve and the varying metrics of success.
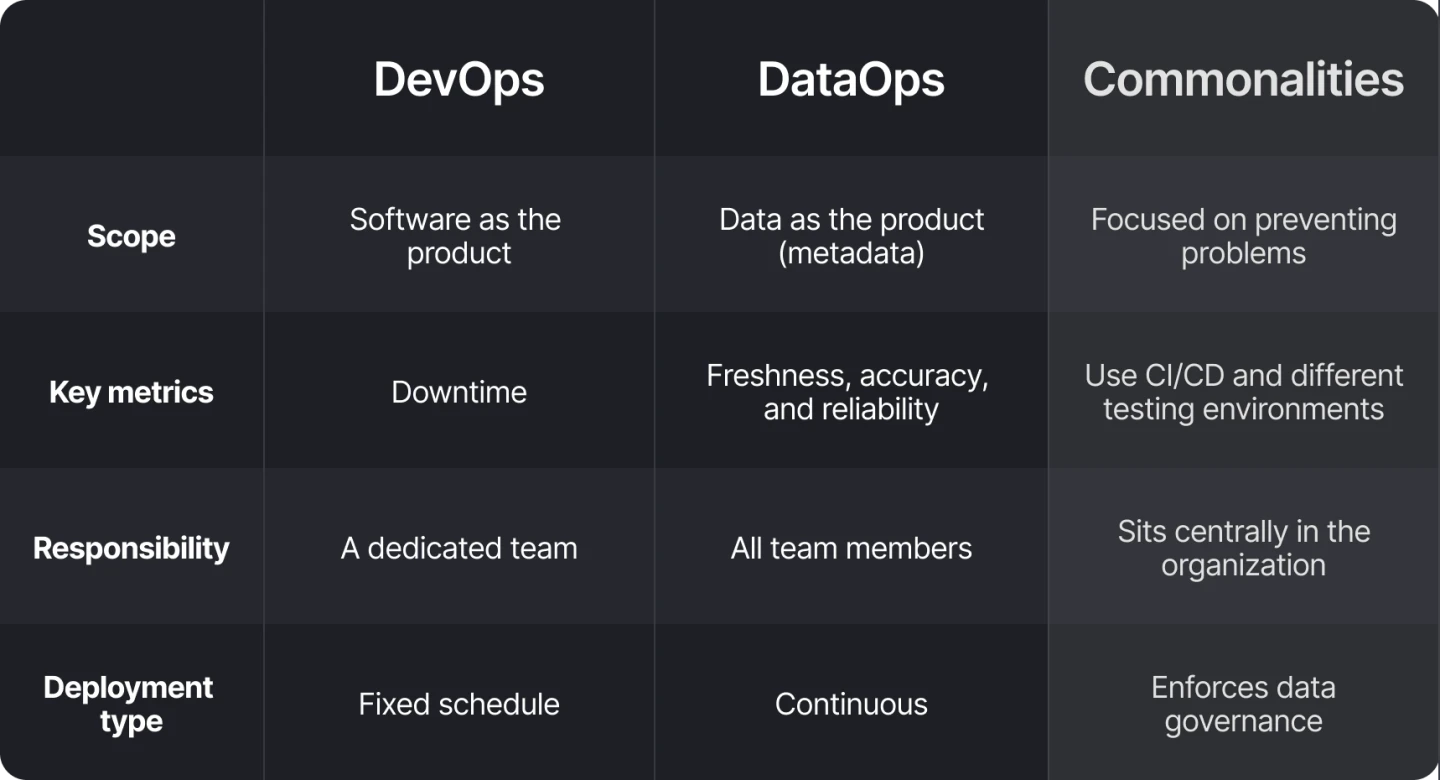
Comparison table: DataOps vs DevOps
What is DevOps?
DevOps involves the deployment and testing of software code changes. When I worked as a DevOps engineer it often involved long deployment nights, testing code changes in many different environments, and validating the changes with the software engineers that made them.
Software as a product
DevOps teams work to serve software engineers. They help to manage the environments where changes are occurring, ensuring downtime is minimized and platforms can scale accordingly. DevOps data engineers don’t write the code they are deploying but rather manage the infrastructure that supports the code.
I would often work with software engineers to determine when changes should be pushed, which environment they should be pushed to, and then to validate that our AWS resources could properly handle the changes. I never read over pull requests or checked how someone’s JavaScript was written. I instead made sure I chose the right cluster size and scaled cloud resources accordingly. It’s important to remember that DevOps specifically focuses on the deliverability of software code changes.
To ensure you can deliver as a DevOps team, you need to have processes in place like building CI/CD pipelines and using these to constantly reiterate. Without proper testing before pushing something to production, deliverability often fails. To prevent breaking code it is critical to proactively test how code manifests in a new environment.
DevOps key metric of success
DevOps teams use downtime as their core measure of success. This was what we checked religiously on my team to see how we were doing compared to previous months, or other teams. If our downtime was increasing, we knew there was something that needed to be changed. It was also a great indicator that the improvements in our systems were working.
If you’ve done your job well, downtime is often limited because everything in the deployment process went smoothly. It signifies that you were properly prepared and knew how to fix potential problems that came your way.
However, if the downtime is long, it’s a good indicator that something went wrong in the deployment process or the system couldn’t scale accordingly with the changes. This is critical in DevOps because customers are typically the ones affected by downtime. This is why you typically see SEV 1 and SEV 2 incidents caused by DevOps teams. These are considered critical because they are preventing customers from using your product.
What is DataOps?
DataOps treats data as the product. There isn’t a core software feature it supports but rather it focuses on high-quality metadata. As an analytics engineer, I focus on DataOps every day. If I don’t produce data that is fresh, accurate, and reliable then what is the point?
Data as a product
DataOps is a core part of any data role. Unlike DevOps, it’s not a separate team that overlooks changes, but rather something every data practitioner must perform. Analytics engineers and data engineers are expected to validate the data models they use in their models and the data their models produce. Many, like myself, do their own testing and then monitor those tests to ensure everything is going smoothly. There isn’t a separate team to quality-check how the new data is integrating into the already existing data environment.
With DataOps, there isn’t necessarily a deployment schedule or process, because it’s ongoing. Analytics and data engineers are expected to constantly monitor databases, data warehouses, and any other systems that generate data. Instead of a rigid process, it’s about continuously making changes and ensuring you test and monitor along the way.
However, like DevOps, DataOps may also involve building out features like CI/CD pipelines in order to make code changes easier and more reliable. DataOps can also involve setting up different environments to test how changes to source data or data models affect data downstream.
DataOps key metric of success
Because DataOps is focused on data quality, key metrics of success include freshness, how close the computed values compare to actual values, and how often the data is available when it is needed. The same metrics that are emphasized in observability are considered metrics of success in DataOps.
At the end of the day, the data that is being produced needs to be used by the business. If the business can’t use the data it needs to make decisions, DataOps isn’t being done well. Its purpose is to guide the business in the direction it needs to succeed and make the most revenue. High-quality data will help you do that, but low-quality data will do quite the opposite.
Conclusion
While DevOps and DataOps are different in the products they serve and the metrics they measure, at the end of the day, both are in place to serve the end user and give them the best experience possible. For DevOps, this is a customer. For DataOps, this is a business stakeholder.
Both DevOps and DataOps focus on the health of their products and gain insights into the possible things that can go wrong. They try to prevent any negative impacts on customers or stakeholders by properly testing the changes they make before they make them. At the core, they exist to minimize the number of problems that a business faces.
While my day-to-day as an analytics engineer and DevOps data engineer are very different, both of my teams sat at the center of their organization. Their goal is to serve others and ensure changes go smoothly. They share common best practices such as enforcing strict data governance within an organization, using version control, and implementing CI/CD. At their core, DevOps and DataOps are service-centered disciplines that many companies would be very lost without.
Y42 as a turnkey DataOps platform
Y42 has been built with the data team in mind and the philosophy of treating data as a product. While it helps data teams orchestrate their data pipelines end-to-end, it provides a monitoring function to continuously observe data pipeline health and give data teams the visibility and control that they need, enabled by the implementation of Stateful Data Assets.
Category
In this article
Share this article